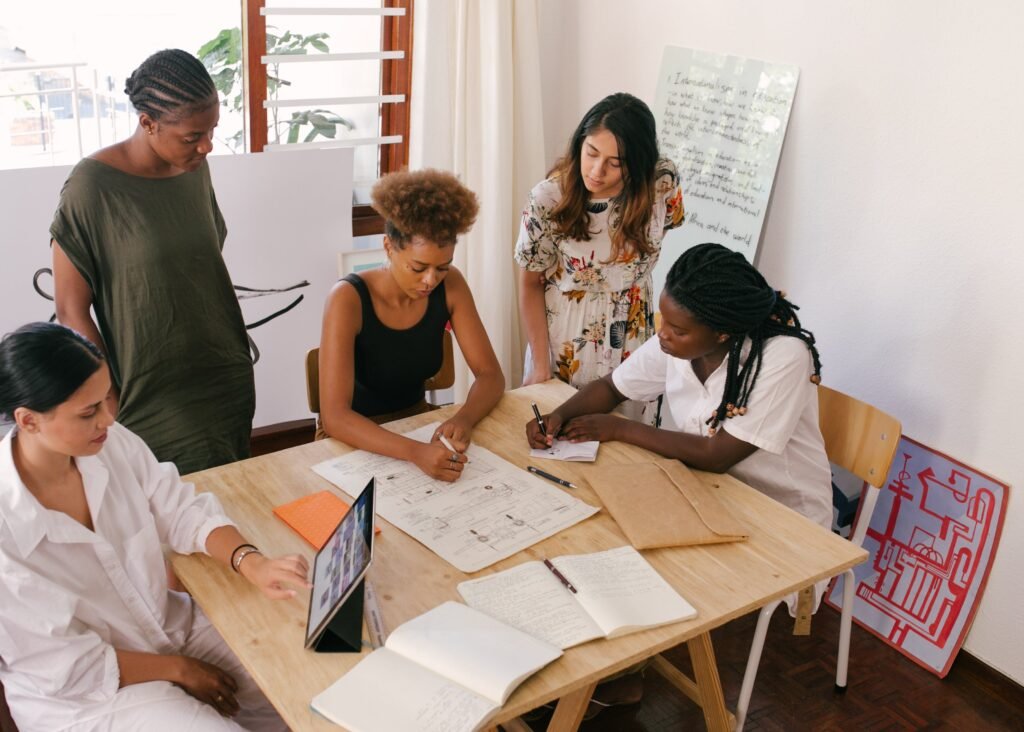
The technological landscape is witnessing a seismic shift towards open-source and decentralized Artificial Intelligence (AI), marking a pivotal chapter in the annals of computer science and, more broadly, in the future of IT and Artificial Intelligence. This movement is not just a page out of science fiction; it is the unfolding narrative of how collective intellect is shaping the theory of mind in machines.
Open Source AI: The Democratization of Machine Intelligence
Open Source AI is reshaping the theory of mind for machines, with developers at the forefront of this revolution. Platforms like GitHub have become the digital agora for AI technology, where generative AI, a subset of artificial general intelligence (AGI), is flourishing. In 2023, GitHub saw generative AI projects make it to the top 10 most popular open source projects by contributor count, indicating a massive 148% year-over-year growth in individual contributions.
The theory of mind, a concept crucial to AI, particularly in understanding and predicting the needs and intentions of users, is being advanced by open source projects. These projects are not confined to the realm of supervised learning; they encompass various types of artificial intelligence, from simple automated responses to complex decision-making systems that mirror human cognition.
The Impact of Generative AI on Development and Economy
The emergence of generative AI has been nothing short of explosive. In 2023 alone, the number of generative AI projects more than doubled compared to 2022. These projects are expanding the boundaries of machine learning and deep learning, bringing AI’s transformative power into everyday applications.
This surge in AI development is not just a technical phenomenon; it’s an economic catalyst. Research in collaboration with Harvard Business School and Keystone.AI suggests that the productivity gains from generative AI could add a staggering $1.5 trillion to the global economy by 2030, along with 15 million effective developers.
Decentralized AI: Nurturing Ethical and Fair Machine Intelligence
Decentralization in AI is a movement toward a more equitable and transparent AI landscape. It involves shared development and the use of AI technology to create tools that are unbiased and adhere to ethical standards. Developers are actively creating and sharing tools for responsible AI, fairness in AI, responsible machine learning, and ethical AI on GitHub.
This commitment to responsible AI development is not just about creating fair algorithms; it’s about passing the Turing test of ethics in AI. Decentralized AI nurtures a community where large language models, such as OpenAI’s GPT, are not only judged on their technical prowess but also on their societal impact and alignment with human values.
Decentralized AI, which involves distributing artificial intelligence (AI) processes across a network of devices or nodes rather than relying on a centralized server, holds great potential for nurturing ethical and fair machine intelligence. This approach addresses several key challenges associated with AI systems, including bias, transparency, privacy, and accountability.
- Reducing Bias: Centralized AI systems can inadvertently perpetuate biases present in their training data or algorithms. Decentralized AI, by involving a diverse set of devices and sources in the decision-making process, has the potential to reduce bias. The input data and perspectives are more varied, leading to a more inclusive and balanced representation.
- Increased Transparency: Decentralized AI systems often involve multiple parties contributing to the learning process. This collaborative nature fosters transparency as each participant can scrutinize and understand the contributions and decisions made by the system. This transparency is crucial for building trust in AI technologies, especially when decisions impact individuals or communities.
- Privacy Protection: Decentralization can enhance privacy by keeping sensitive data localized and reducing the need for central repositories. In a decentralized AI network, models can be trained on user devices without the need to share raw data. This protects user privacy while still allowing the model to learn and improve.
- Distributed Accountability: Traditional centralized AI systems often lack clear lines of accountability when things go wrong. In decentralized AI, responsibility is distributed across the network. This can make it easier to trace issues back to their source, facilitating accountability and ensuring that those responsible for problems can be identified and held accountable.
- Robustness and Resilience: Decentralized systems are inherently more resilient to failures. If one node in the network fails, others can continue to function independently. This can help prevent catastrophic failures and enhance the overall robustness of AI systems.
- Empowering Local Communities: Decentralized AI empowers local communities by allowing them to participate in and benefit from AI development. This can lead to the creation of AI models that better serve the specific needs and nuances of different communities, promoting inclusivity and avoiding one-size-fits-all solutions.
- Open Source Collaboration: Decentralized AI often involves open-source collaboration, enabling a wider community of researchers, developers, and ethicists to contribute to the development of AI models. This collaborative approach fosters a shared commitment to ethical practices and encourages the adoption of ethical guidelines in the development process.
- Regulatory Compliance: Decentralized AI systems can facilitate compliance with data protection regulations, as they often involve processing data on local devices rather than central servers. This aligns with principles of data minimization and user control over personal information.
AI’s Role in the Evolution of Computer Science
Artificial Intelligence (AI) plays a pivotal role in shaping the evolution of computer science in numerous ways. It not only represents a specialized field within computer science but also acts as a catalyst for advancements and transformations across the entire discipline. Here are several key aspects highlighting AI’s influence on the evolution of computer science:
- Foundations of AI as a Subfield: AI emerged as a subfield of computer science, focusing on the development of intelligent agents capable of perceiving their environment and making rational decisions. The quest to create machines that mimic human intelligence has driven research in areas such as machine learning, natural language processing, robotics, and expert systems.
- Machine Learning Paradigm Shift: AI has driven a paradigm shift in machine learning, a subfield that focuses on developing algorithms that enable computers to learn from data. The intersection of AI and machine learning has led to breakthroughs in pattern recognition, predictive modeling, and decision-making. Deep learning, a subset of machine learning, has gained prominence with neural networks capable of complex tasks like image recognition and natural language understanding.
- Automation and Optimization: AI has significantly impacted computer science by automating routine and labor-intensive tasks. Automation, facilitated by AI algorithms, has improved efficiency and productivity across various domains. Machine learning algorithms are increasingly used for optimization problems, enhancing resource allocation, scheduling, and decision-making processes.
- Natural Language Processing (NLP): AI’s contributions to natural language processing have transformed the way computers interact with humans. NLP enables machines to understand, interpret, and generate human language, leading to applications such as virtual assistants, chatbots, and sentiment analysis. This has profound implications for human-computer interaction and information retrieval.
- Computer Vision and Image Recognition: AI has revolutionized computer vision, allowing machines to interpret and understand visual information. Image recognition and object detection, powered by AI algorithms, find applications in areas like autonomous vehicles, medical image analysis, and surveillance systems.
- AI in Robotics: The integration of AI with robotics has opened up new possibilities in automation, autonomous navigation, and human-robot interaction. Intelligent robots, equipped with machine learning algorithms, can adapt to changing environments, learn from experience, and perform complex tasks.
- Ethical and Social Implications: AI has brought ethical considerations to the forefront of computer science. As AI systems influence decision-making in critical areas such as healthcare, finance, and criminal justice, computer scientists are actively addressing issues related to bias, transparency, accountability, and the societal impact of AI technologies.
- Interdisciplinary Collaboration: AI’s interdisciplinary nature has fostered collaboration between computer scientists and experts from diverse fields, including neuroscience, psychology, linguistics, and ethics. This interdisciplinary approach has enriched the study of AI and expanded its applications.
- AI and Data Science Integration: The rise of AI has closely paralleled the growth of data science. AI algorithms leverage vast amounts of data to train models, and the synergy between AI and data science has led to innovations in predictive analytics, recommendation systems, and personalized services.
- Evolution of Computing Architectures: The demand for more powerful computing resources to train and deploy AI models has driven advancements in computing architectures. Graphics Processing Units (GPUs) and specialized hardware accelerators are increasingly used to handle the computational intensity of deep learning algorithms.
The Future of AI and its Integration with Daily Life
The future of IT and artificial intelligence is one where AI permeates every aspect of our lives. From driving cars to large language models assisting in real-time language translation, AI’s integration into daily life is becoming seamless. The ongoing development of deep neural networks promises to make AI not just a tool but a companion in the human journey.
In conclusion, the growth of open-source and decentralized AI heralds a new dawn for artificial intelligence AI, where the collective contributions of developers are driving the future of IT and artificial intelligence. This collaborative effort in advancing AI technology is not only pushing the boundaries of computer science but also ensuring that the fabric of AI is woven with the threads of diversity, ethics, and inclusivity. As we continue to train our machine learning models and enhance our deep neural networks, it is essential to remember that the true power of AI lies in our ability to harness it for the greater good, a principle that stands at the core of UpskillYourself’s mission to educate and empower the AI professionals of tomorrow.