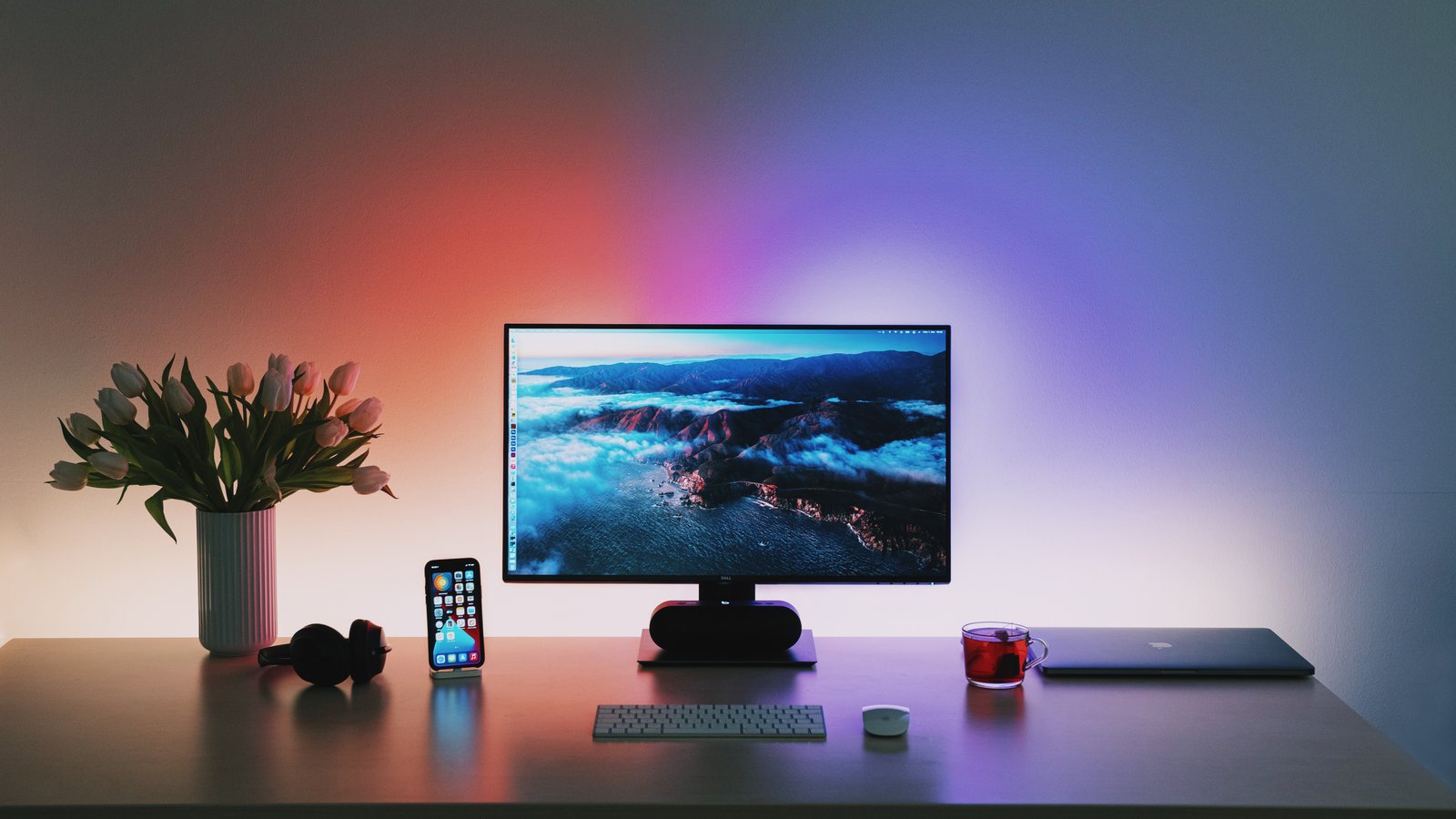
Artificial Intelligence (AI) and Machine Learning (ML) have emerged as transformative technologies, promising to revolutionize industries, automate processes, and enhance decision-making. However, beneath their potential lies a complex ethical landscape, particularly when it comes to bias and fairness in AI and ML models. In this comprehensive 1500-word exploration, we will delve deep into the ethical considerations surrounding AI, dissect the sources of bias within ML models, examine the real-world ramifications of biased AI, and outline effective strategies to mitigate bias and promote fairness.
The Ascension of AI and ML
The past decade has witnessed the meteoric rise of AI and ML. These technologies have infiltrated every facet of modern life, from healthcare and finance to entertainment and transportation. They hold the promise of improving efficiency, predicting outcomes with remarkable accuracy, and personalizing user experiences. Yet, with these promises come significant challenges, and chief among them is the pressing issue of bias and fairness.
Deciphering Bias in AI
As artificial intelligence (AI) continues to play an increasingly prominent role in various aspects of our lives, concerns about bias in AI systems have gained significant attention. Bias in AI refers to the presence of prejudiced or unfair outcomes in the decision-making process of machine learning models. Deciphering bias in AI is a multifaceted challenge that involves understanding its origins, impacts, and the ongoing efforts to address and mitigate it.
Understanding Bias in AI:
- Data Bias: Bias often originates from the training data used to develop AI models. If the training data is not diverse or representative, the model may learn and perpetuate the biases present in that data.
- Algorithmic Bias: The design and optimization of algorithms can introduce bias. Biased decision-making criteria, unintended correlations, or inadequate model evaluation processes contribute to algorithmic bias.
Types of Bias in AI:
- Selection Bias: Arises when the training data is not representative of the broader population, leading the model to make biased predictions for certain groups.
- Labeling Bias: Occurs when the data used for model training is labeled with biased or subjective annotations, influencing the model’s understanding.
- Algorithmic Bias: Results from the design and coding of algorithms, where the decision-making process inherently favors or disadvantages certain groups.
Impacts of Bias in AI:
- Unfair Treatment: Biased AI systems can lead to unfair treatment of individuals or groups based on factors such as race, gender, or socioeconomic status.
- Reinforcement of Stereotypes: Biased models may reinforce existing societal stereotypes, perpetuating harmful and inaccurate representations.
- Discriminatory Outcomes: Bias in AI can result in discriminatory outcomes, affecting decisions in areas such as hiring, lending, and law enforcement.
Detecting Bias in AI:
- Auditing and Testing: Regularly audit and test AI models for bias using diverse datasets. Assess the model’s performance across different demographic groups to identify disparities.
- Explainability: Implement explainable AI techniques to understand how models arrive at specific decisions. This transparency aids in identifying and addressing biased patterns.
- Diversity in Development: Foster diversity in AI development teams to bring a range of perspectives and experiences, reducing the likelihood of biased design and decision-making.
Addressing and Mitigating Bias:
- Ethical Guidelines: Establish and adhere to ethical guidelines in AI development, emphasizing fairness, transparency, and accountability.
- Diverse Training Data: Ensure training datasets are diverse, representative, and free from inherent biases. This includes careful curation and continuous monitoring.
- Algorithmic Fairness Techniques: Explore and implement algorithmic fairness techniques that aim to mitigate bias and ensure equitable outcomes.
- Bias Detection Tools: Utilize specialized tools and frameworks designed to detect and quantify bias in AI models.
Ethical Considerations:
- User Consent and Transparency: Inform users about the use of AI systems and obtain their consent. Transparent communication about how AI decisions are made fosters trust.
- Bias Correction vs. Model Accuracy: Consider the trade-off between correcting bias and maintaining model accuracy. Striking a balance is essential for fair and effective AI systems.
Continuous Improvement:
- Feedback Loops: Establish feedback loops for continuous improvement. Act on feedback from users and affected communities to refine models and reduce biases over time.
The Implications of Bias in AI
Unfair Treatment:
- Implication: AI systems that exhibit bias may lead to unfair treatment of individuals or groups based on characteristics such as race, gender, or socioeconomic status.
- Impact: Unfair treatment perpetuates social inequalities and undermines the principles of equal opportunity and justice.
Reinforcement of Stereotypes:
- Implication: Biased AI models may perpetuate and reinforce existing societal stereotypes by learning and replicating patterns present in training data.
- Impact: This reinforcement contributes to the perpetuation of harmful and inaccurate stereotypes, reinforcing prejudices and discrimination.
Discriminatory Outcomes:
- Implication: Bias in AI can result in discriminatory outcomes in critical areas such as hiring, lending, and criminal justice.
- Impact: Discriminatory outcomes contribute to systemic injustices, exacerbating existing societal disparities and leading to negative consequences for affected individuals.
Erosion of Trust:
- Implication: Discovering biased behavior in AI systems erodes public trust in these technologies and the organizations deploying them.
- Impact: Reduced trust can hinder the widespread adoption of AI solutions and undermine the potential benefits these technologies offer.
Unintended Consequences:
- Implication: Unintended consequences of biased AI decisions can lead to unforeseen negative impacts on individuals and communities.
- Impact: These unintended consequences may create social unrest, legal challenges, and ethical dilemmas, further complicating the deployment of AI technologies.
Exacerbation of Inequities:
- Implication: If not properly addressed, biased AI systems can exacerbate existing societal inequities and widen the gap between privileged and marginalized communities.
- Impact: The exacerbation of inequities further marginalizes vulnerable populations, perpetuating a cycle of disadvantage.
Negative Impact on Innovation:
- Implication: Bias in AI can stifle innovation by limiting opportunities for individuals and groups who are disproportionately affected by biased algorithms.
- Impact: The exclusion of diverse perspectives and talents hampers creativity and innovation, hindering the development of inclusive and beneficial AI solutions.
Legal and Ethical Challenges:
- Implication: The presence of bias in AI systems raises legal and ethical challenges, with potential consequences for organizations responsible for deploying biased technologies.
- Impact: Legal challenges can result in regulatory scrutiny, fines, and reputational damage, emphasizing the need for ethical considerations in AI development.
Public Backlash:
- Implication: Instances of biased AI decision-making can lead to public backlash, causing individuals and communities to reject or resist the adoption of AI technologies.
- Impact: Public resistance can impede the positive impact that AI can have on society, hindering its potential for addressing complex challenges.
Undermining Diversity and Inclusion:
- Implication: Bias in AI can undermine efforts to promote diversity and inclusion by perpetuating systemic biases and limiting opportunities for underrepresented groups.
- Impact: The exclusion of diverse perspectives hampers the development of fair and inclusive AI systems, limiting their effectiveness and societal benefits.
In summary, the implications of bias in AI are multifaceted and encompass social, ethical, legal, and technological dimensions. Addressing bias requires a concerted effort from developers, researchers, policymakers, and society as a whole to ensure that AI systems are fair, transparent, and aligned with ethical principles. Recognizing and mitigating bias is a critical step toward unlocking the full potential of AI for the benefit of humanity.
Navigating Bias: Strategies for Fairness
Addressing bias in artificial intelligence (AI) systems is crucial to ensure fairness, equity, and ethical use of these technologies. Navigating bias requires a combination of thoughtful strategies at various stages of the AI development lifecycle. Here are key strategies for promoting fairness in AI:
Diverse and Representative Training Data:
- Strategy: Curate diverse and representative datasets that encompass a wide range of demographic groups and scenarios.
- Rationale: Training AI models on diverse data helps mitigate biases by exposing the system to a comprehensive set of examples, reducing the risk of skewed representations.
Bias Detection and Assessment:
- Strategy: Implement robust bias detection tools and metrics to continuously assess model performance across different demographic groups.
- Rationale: Regularly evaluating AI models for bias allows developers to identify and rectify potential issues before deployment, ensuring fairness in outcomes.
Explainability and Transparency:
- Strategy: Design AI models to provide explainable outputs, allowing users to understand how decisions are made.
- Rationale: Transparent AI systems foster trust and enable stakeholders to comprehend and challenge decisions, reducing the likelihood of biased outcomes going unnoticed.
Fairness Metrics and Evaluation:
- Strategy: Define fairness metrics tailored to the specific context and goals of the AI system. Evaluate models using these metrics.
- Rationale: Customized fairness metrics help quantify and measure fairness, providing insights into potential disparities in outcomes.
Algorithmic Fairness Techniques:
- Strategy: Implement algorithmic fairness techniques, such as reweighting, re-sampling, and adversarial training, to mitigate biases in model predictions.
- Rationale: These techniques adjust the learning process to address disparities, promoting fairness in the model’s decision-making.
Diversity in Development Teams:
- Strategy: Foster diversity in AI development teams to bring varied perspectives and experiences.
- Rationale: Diverse teams are more likely to identify and mitigate biases during the development process, ensuring a broader understanding of potential impacts.
User Feedback and Participation:
- Strategy: Encourage user feedback and participation in the development and testing phases of AI systems.
- Rationale: Direct input from users, especially those from diverse backgrounds, helps identify biased outcomes and contributes to refining models for fairness.
Ethical Guidelines and Governance:
- Strategy: Establish and adhere to ethical guidelines for AI development. Implement governance structures to ensure compliance with fairness principles.
- Rationale: Ethical guidelines provide a framework for responsible AI development, guiding decisions and actions to prioritize fairness and ethical considerations.
Continuous Monitoring and Iterative Improvement:
- Strategy: Implement continuous monitoring of AI models post-deployment, and iterate on models to improve fairness.
- Rationale: Ongoing monitoring allows for the identification of emerging biases and provides opportunities to refine models based on real-world performance.
Educational Initiatives:
- Strategy: Promote education and awareness about bias in AI among developers, stakeholders, and the wider community.
- Rationale: Increased awareness fosters a proactive approach to addressing bias, encouraging a collective commitment to fairness in AI development.
Collaboration and Knowledge Sharing:
- Strategy: Encourage collaboration and knowledge sharing within the AI community to exchange best practices for mitigating bias.
- Rationale: A collaborative approach enables the collective wisdom of the AI community to address challenges and implement effective strategies for fairness.
Human-in-the-Loop Approaches:
- Strategy: Integrate human-in-the-loop approaches to involve human oversight in critical decision-making processes.
- Rationale: Human oversight adds a layer of contextual understanding and ethical judgment, helping prevent and correct biased outcomes.
By combining these strategies, developers, researchers, and organizations can actively work towards creating AI systems that prioritize fairness, minimize bias, and contribute to a more equitable and inclusive technological landscape. It requires a commitment to ongoing learning, collaboration, and a shared responsibility to navigate bias and ensure the responsible deployment of AI technologies.
Real-World Examples
Let’s explore a few real-world examples that vividly illustrate the critical importance of addressing bias and promoting fairness in AI:
1. Biased Facial Recognition
Facial recognition technology has come under scrutiny for bias, particularly in its accuracy across different racial groups. Biased facial recognition can lead to false identifications and unjust arrests, highlighting the life-altering consequences of biased AI.
2. Algorithmic Hiring Bias
Several companies have faced allegations of algorithmic bias in their hiring processes. Biased AI systems can discriminate against candidates based on gender, age, or ethnicity, perpetuating inequalities in the job market.
3. Healthcare Algorithms
In healthcare, AI algorithms have been criticized for being less accurate for certain demographic groups. For example, a skin cancer detection AI may perform less effectively on people with darker skin tones, potentially delaying diagnoses and treatments.
Charting the Path Forward
As AI and ML continue their relentless march of progress, addressing bias and promoting fairness must be at the forefront of technological development. Ethical AI practices, diverse and unbiased datasets, transparency in model development, continuous monitoring, and adherence to ethical guidelines are essential steps in ensuring that these technologies serve the greater good without perpetuating discrimination.
Conclusion
In conclusion, ethical AI stands as a pressing and complex concern that demands our attention and concerted action. The presence of bias and the pursuit of fairness in machine learning models have profound implications for individuals and society at large. By acknowledging the sources of bias, implementing best practices, fostering transparency, and embracing ethical guidelines, we can collectively create AI systems that are not only technically proficient but also fair, equitable, and beneficial for everyone. As we navigate the uncharted waters of the AI revolution, the ethical imperative of addressing bias and promoting fairness cannot be overstated. It is a collective responsibility to ensure that AI serves as a force for good, enriching rather than exacerbating the complexities of human existence in our increasingly digital world.